Advances in drug development and discovery have revolutionized the delivery of life-saving treatments to patients. Despite the immense impact of these innovations, the journey from concept to bedside remains largely misunderstood. Through this primer series, we explore the complex processes behind drug discovery, highlighting the challenges the industry still faces and the critical need for investment of time and resources to deliver the next generation of medicines.
September 2024
Introduction
Luma Group’s mission is to invest in the most innovative companies poised to make meaningful impacts on human health outcomes. We focus on therapeutics, diagnostics, and medical devices because they provide a unique opportunity for venture capital to thrive, while fueling innovation that will benefit humanity. Despite the enormous impact that medical improvements have had on nearly all lives, the process to develop these drugs (”drug discovery”) remains poorly understood by most due to its long, arduous, expensive and highly complex nature.
We seek to shed light on this topic, and several others in our primer series. Through these primers, we hope to provide additional clarity into several integral processes underlying the biotech industry to help a broader understanding of the challenges the industry faces and the necessity for continued investing here. We hope readers walk away with a deeper appreciation and a clearer understanding of the immense and collaborative effort involved in the biotechnology community’s journey to advance drug discovery and development. It truly takes a village to create a drug.
In part one of this primer series, we will explore the various steps of the drug discovery process, delving into the key elements of each step and the challenges and opportunities for future growth (Figure 1). Despite the inherent complexities and obstacles, we are in the golden age of innovation within our sector. Our goal is to help demystify the journey of how drugs progress from the laboratory bench to the patient’s bedside.
In this document, we break down the drug discovery process into distinct steps and summarize each with a table that reviews key aspects. These aspects include the step’s “significance,” which refers to its importance in the overall process of creating a drug; its “purpose,” highlighting the key data generation objectives; its “probability of in-stage success (PoS),” indicating the likelihood that a drug candidate will successfully advance to the next stage; its “timelines/costs,” which detail the expected duration and associated expenses; and its “areas for optimization,” where we identify opportunities for companies to enhance their risk-adjusted outcomes in drug discovery.
To supplement this series and other Luma content, we have compiled a target glossary of key technical terminology to support readers. This glossary can be accessed here.
For every 100 candidates entering the drug discovery process, it is estimated that only between 1 to 3 will ultimately become FDA-approved drugs that reach commercialization. However, it is important to note that in the earliest stages of development, such as compound screening, depending on the methodology used, a group may need to screen tens of thousands of compounds just to find a single hit. This reality underscores the challenge of quantifying asset attrition, and the graphic below serves to illustrate the monumental undertaking required to get a new drug to patients.
Figure 1: Overview of critical endpoints, cost, and risk profile of each discovery and development stages.
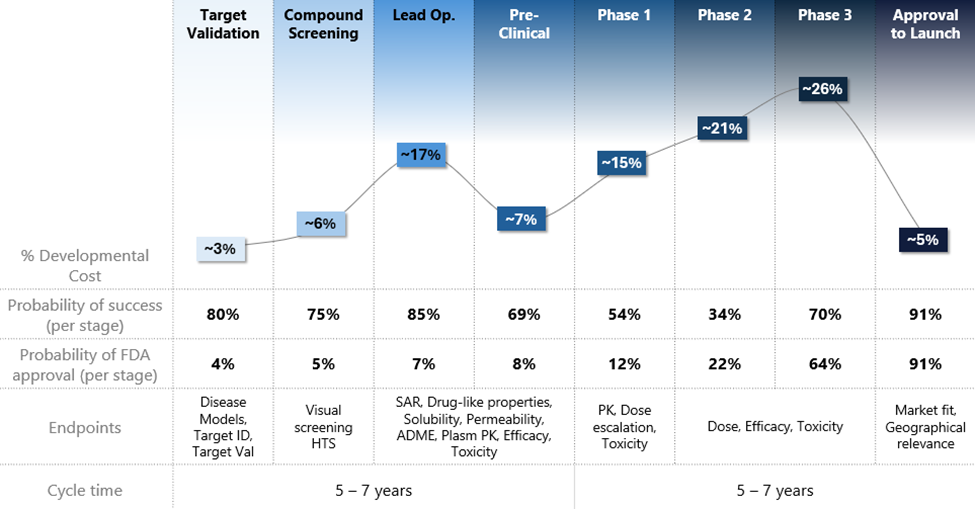
Asset counts beings after developmental candidate nomination as after this point a drug format has been finalized for development and failure becomes a binary process. Source: Deal Room, Speedinvest.
Importance of Drug Discovery
Modern medicine has revolutionized billions of lives, from using antibiotics to combat once-deadly bacterial infections to developing cures for life-threatening genetic diseases. Although only a few drugs ultimately reach the hands of physicians, countless hours and numerous resources are poured into these efforts of discovering and developing the next generation of life-saving drugs. The entire journey – from taking a new biological discovery in a university lab to eventually saving a patient’s life – is what we think of as “drug discovery”.
Figure 2: Key steps in the drug development process.
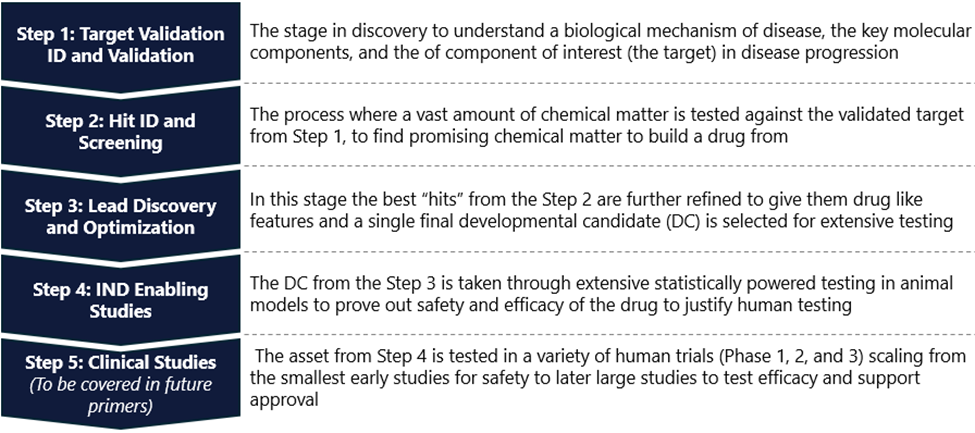
Source: Luma proprietary.
Early pioneers in drug discovery laid the foundation for our modern drug discovery process. Visionaries like Louis Pasteur, who pioneered the germ theory of disease, Alexander Fleming, who discovered penicillin, Jonas Salk, who developed the polio vaccine, and Dorothy Hodgkin, who unlocked the structures of important biochemical substances, dedicated their lives to transforming their scientific breakthroughs into what we now recognize as life-saving drugs.
Their work has inspired countless researchers to convert their own insights into new therapies that continue to save lives. In today’s landscape, regulators play a crucial role in drug discovery, and understanding their influence is essential to grasping the complexities of the process.
Over the past 120 years, the FDA in the US and the EMA in the EU have standardized the drug approval process, ensuring that only the safest drugs and most effective drugs reach the market. The rigorous standards set by these regulatory bodies have established best practices across the industry, even influencing other jurisdictions, thereby accelerating the timeline from discovery to approval and significantly increasing the efficiency of approvals. However, while this robust regulatory framework has positive outcomes, it is lengthy and costly, typically requiring $350 million and 10-15 years to bring a promising discovery to the point of commercial (i.e., patient care).1 Navigating this complex process demands both experience and substantial capital, critical resources provided by venture capital investors to the innovative scientists driving discovery.
The global drug market has seen remarkable and sustained growth over the last 50 years, expanding from around $12 billion in the 1970s to over $1.56 trillion by 2020.2 This tremendous growth, driven by the development of new therapies and the expansion of global markets, is expected to continue at approximate 6% compound annual growth rates, potentially reaching nearly $3 trillion within the next decade.3
This growth is driven by advancements in biotechnology, increased investment in research and development and the introduction of innovative therapies for chronic and complex diseases. For instance – the development of biologics, such as monoclonal antibodies and gene therapies, has revolutionized treatment options for conditions like cancer, rheumatoid arthritis, and genetic disorders.
The success of drugs such as Humira, one of the world’s best-selling drug (over ~$20B in sales/year), illustrates the potential for growth and impact in the pharmaceutical industry. Additionally, the rapid development and deployment of COVID-19 vaccines demonstrated the industry’s and regulator’s capacity for swift innovation and large-scale production in response to global health crises.
The Long Journey of Drug Discovery and Development
Step 1. Target Identification and Validation in Drug Discovery
Target identification and validation is the first step in the extensive drug discovery and development process. This crucial phase involves selecting a specific node within a disease pathway to target with a new drug. Typically, this process originates from foundational research conducted in academic labs, where scientists strive to understand the underlying biology of specific diseases. For example, researchers may find a protein or molecule crucial to disease progression (the “target”).
This foundational knowledge is the springboard for drug development – the earliest stage. Successfully transitioning this early-stage academic research into a plausible biotech venture often hinges on validating the target – expanding on the academic research that’s already been completed. See “Validating Drug Targets” below for a further explanation.
Identifying Drug Targets
Identification can take a top-down (“clinical sample”) or bottom-up (“research sample”) approach for identification. The top-down approach typically looks for causal relationships of a target from a patient sample and requires basic research to validate the causal nature. For instance, in breast cancer research, scientists took a clinical top-down approach to identify the HER2 protein, which is overexpressed in a subset of breast cancer tumors. This discovery was pivotal as it led to the development of targeted therapies like trastuzumab (Herceptin), which specifically inhibits HER2, thereby slowing tumor growth and improving patient outcomes.
The bottom-up approach aims to perturb specific target biology and look for outcomes reflecting patient disease pathology (Figure 3). Paclitaxel, a pioneering chemotherapeutic agent, was discovered through natural product research. Scientists at the US National Cancer Institute identified a compound in the Pacific yew tree (Taxus brevifolia) that inhibited cancer cell growth in cellular models. Later synthesized in laboratories to enhance production efficiency, this discovery has profoundly advanced the treatment of several cancers, including ovarian and breast cancer.4
The clinical sample approach has progressively become the first choice in drug development. By starting with patient samples from the target disease, it can help researchers identify mutations or aberrations only present in the diseased patients and provide a narrowed starting universe for testing. In the bottoms-up approach, discovery relies more heavily on trial and error as there isn’t as robust of an underlying hypothesis around potential disease drivers. However, once the target has been identified and validated, both paths converge on the same output to be taken forward into further stages of development.
Figure 3: Target identification and characterization workflow.
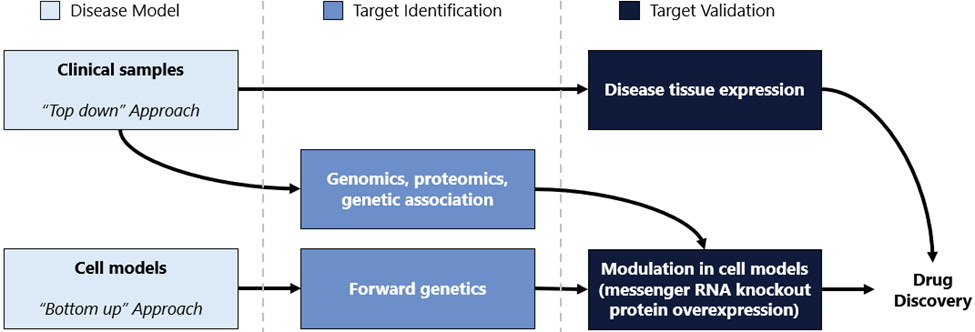
Source: Nature.
Validating Drug Targets
Once a potential target is identified, its role in the disease must be validated to ensure it is a viable candidate for drug development. This is where advanced techniques like “knockout mice” and CRISPR technology come into play.
Knockout mice are mice that are genetically engineered to lack a specific gene – generally the gene associated with the “target,” allowing researchers to observe the effects of its absence on disease progression. This method both offers more data to evaluate the target and helps to establish whether targeting the relevant gene can alter the disease’s course.
CRISPR technology has revolutionized target validation by enabling precise gene editing. This pioneering work has made it significantly easier and cheaper to manipulate multiple genes in cellular and animal models. Researchers can selectively disrupt or modify genes in cell lines or animal models to study their function and contribution to disease. This precise control can provide compelling evidence of a target’s relevance and potential as a therapeutic focus.
Real-World Example: Bridging Academia and Industry
To progress in the drug discovery process, an academic team generally needs to be brought into a commercial environment for capital formation. Spinning out academic research into the pharmaceutical industry requires strategic partnerships, and universities and research institutions often collaborate with biotech companies beginning at this stage. The large academic centers maintain specialized staff who are experienced in creating these collaborations in a way that rewards the university (or other institution) to the extent the commercialization effort is successful. A large part of our opportunities at Luma Group come from this nexus.
Key Takeaways for Step I
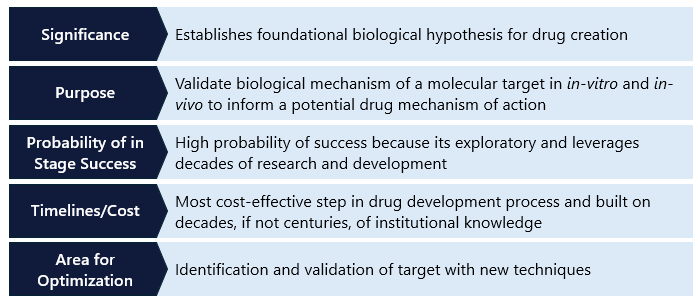
Step 2. Hit Identification and Screening in Drug Discovery
The next pivotal phase in drug discovery after target identification and validation is hit identification and screening. This stage involves finding compounds that can interact with the identified target, which may lead to a potential therapeutic effect. This step typically involves screening thousands to billions of compounds to identify the roughly <0.5% that interact with the target. The goal is to identify those that show some level of activity – these are referred to as “hits” and require further refinement and optimization. This is an iterative cycle, where researchers use the early results to increase the chances of finding more hits. Some of these hits will be taken forward as candidates and some may serve as backups or future “next generation” assets against the same target. Different strategies can be employed in hit identification and screening, which can provide unique advantages or differentiation in the drug discovery process. We will only cover the most common strategies in this primer.
High-Throughput Screening (HTS)
High-Throughput Screening (HTS) is a crucial and powerful tool in drug discovery that leverages automation to rapidly screen large libraries of compounds – ranging from thousands to millions – against a specific biological target. By utilizing advanced robotic systems and automated processes, HTS allows for the simultaneous execution of numerous biochemical, genetic or pharmacological assays, significantly accelerating the identification of active compounds, antibodies or genes that influence particular biomolecular pathways. One of the key advantages of HTS is its effectiveness in identifying inhibitors of kinases – a class of enzymatic proteins that play pivotal roles in regulating various cellular processes. Given their importance in disease pathways, kinases are prime targets for HTS, which can efficiently sift through extensive compound libraries to pinpoint molecules that inhibit these critical proteins. These libraries, which may be commercially available, proprietary, or a combination of both, typically contain thousands to billions of compounds.
Despite its broad utility, HTS has limitations. It is less effective when applied to smaller compound libraries, or when the target has limited biological reagents, such as cell-free recombinant proteins, or when structural information about the target is minimal. Despite these challenges, HTS remains a cornerstone of the drug discovery process by identifying promising hits for further development.
Alternative Approaches to Hit Identification
While HTS remains a fundamental method in modern drug discovery, alternative approaches are often necessary, especially when HTS is either impractical or inefficient. For target proteins with poorly understood biology or those from challenging non-kinase classes (such as transcription factors and cell membrane proteins), these alternative strategies may be more successful in generating early-stage drug-like compounds.
- Fragment-Based Drug Discovery (FBDD): Fragment-Based Drug Design involves identifying small chemical fragments that bind to a target protein using a hybrid HTS approach that leverages protein structural information. These fragments are typically simpler than traditional drug molecules, making analyzing their interactions with the target easier. Once a fragment is identified, medicinal chemists build on it, adding functional groups to enhance its binding affinity and specificity. While FBDD can address some of the limitations of traditional HTS, developing fragments into drug-like scaffolds requires significantly more effort. This process demands a substantial amount of structural information to guide the design and optimization. Despite these challenges, FBDD enables the creation of highly optimized drug candidates from small, initially weak-binding fragments.
- Virtual Screening: Virtual screening leverages advanced computer models to predict which compounds are more likely to bind to a target, thereby eliminating the need for labor-intensive wet-lab based HTS methods. This approach simulates interactions between the target and potential drug molecules in silico, enabling researchers to prioritize compounds before any experimental testing. By exploring much larger compound libraries and focusing on the most promising candidates, virtual screening significantly reduces the time and cost associated with hit identification, streamlining the process for subsequent wet lab testing.
Although this approach can save much time and effort compared to manual HTS, it generally yields less reliable results. This is mainly due to limitations inherent in in silico approaches, such as a lack of atomic-level detail, unpredictable outcomes and challenges associated with modeling complex biological systems. While virtual screening is a powerful tool, it is regarded more as a supplementary method than a primary one. It will require further advancements before transitioning to a reliable and widely used primary screening method.
Real-World Example: Discovery of Gleevec (Imatinib)
A notable success story in hit identification through HTS is the discovery of Gleevec (imatinib), a groundbreaking treatment for chronic myeloid leukemia (CML). Researchers identified the BCR-ABL fusion protein as a key driver of CML. Using HTS, they screened hundreds of thousands of compounds to find inhibitors of this protein.5 Imatinib emerged as a potent and specific inhibitor of BCR-ABL, leading to its development and subsequent approval by the FDA. Gleevec has transformed CML from a fatal disease into a manageable condition for many patients, showcasing the power of HTS in drug discovery.
Key Takeaways for Stage II
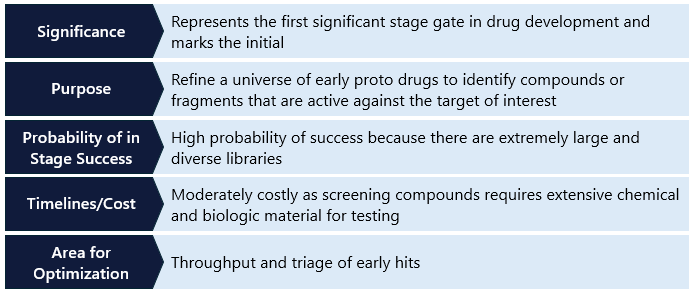
Step 3. Lead Discovery and Optimization in Drug Development
The next critical phase is lead discovery and optimization after identifying potential hits in the drug discovery process. This stage focuses on picking one or more candidate compounds and then refining and improving those compounds to meet specific criteria, ensuring they possess the necessary properties to become effective drugs. Lead compounds are characterized by their potency, selectivity, and overall drug-like properties (see “Criteria for Lead Compounds,” below). This critical step can be one of the longer steps in the process, as development teams make minor modifications to the compound or compounds and repeatedly test those changes to develop stronger candidates for further development (figure 4). It would not be unusual for a team to test hundreds – or even thousands – of potential modifications before settling on a lead candidate.
Criteria for Lead Compounds
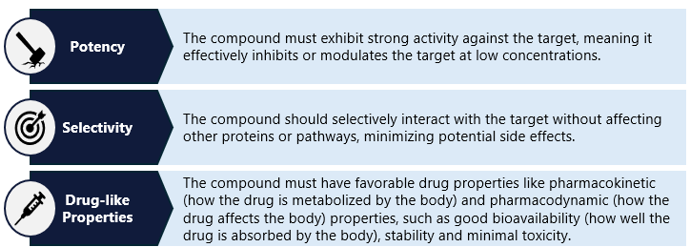
Optimization Strategies
Once lead compounds are identified, they undergo extensive optimization to enhance their properties and maximize their therapeutic potential. This crucial step in drug development involves refining the compounds’ efficacy, safety, and pharmacokinetic properties. While minor optimizations may occur later steps, this initial optimization phase is essential for defining the core characteristics and bulk properties of the drug.
Structure-Activity Relationship (SAR) Studies: SAR studies involve systematically modifying the chemical structure of lead compounds to understand the relationship between their structure and biological activity. By making small changes to the molecule, researchers can determine which modifications improve potency, selectivity, or other desirable characteristics. This iterative process helps fine-tune the compound to achieve optimal performance.
Medicinal Chemistry: Medicinal chemists play a crucial role in optimizing lead compounds. They use their expertise to design and synthesize new analogs, aiming to improve bioavailability, reduce toxicity, and enhance overall drug-like properties. This often involves addressing issues such as poor solubility, metabolic stability, or undesirable pharmacokinetics. Through medicinal chemistry, compounds can be modified to ensure they are suitable for further development.
Figure 4: Workflow for the iterative lead optimization process.
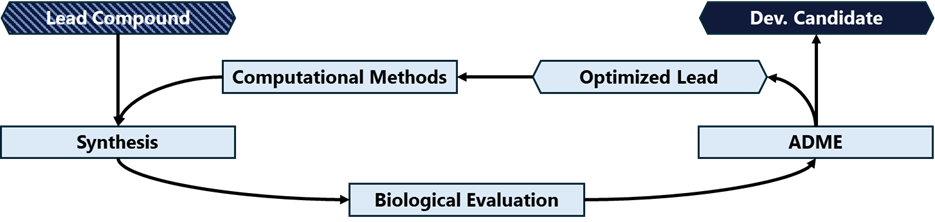
Source: Nature.
Real-World Example: Optimization of HIV Protease Inhibitors
A prime example of successful lead optimization is the development of HIV protease inhibitors. Initially, researchers identified compounds that could inhibit the HIV protease enzyme, which is essential for the maturation and replication of the virus. However, early inhibitors had limitations in terms of potency, bioavailability, and side effects. Through extensive SAR studies and medicinal chemistry efforts, scientists were able to optimize these inhibitors. They made structural modifications in the compound’s p2 and P2” region that binds in the enzymatic domain of the HIV proteases, enhancing potency against drug-resistant variants.6 Additionally, they addressed issues related to bioavailability and metabolic stability, resulting in compounds that were more effective and had fewer side effects. For instance, the optimization of saquinavir, the first HIV protease inhibitor approved by the FDA, involved modifying its chemical structure to improve its pharmacokinetic profile. Researchers at Roche achieved these enhancements using computer-aided SAR and structure-based drug design.7 Subsequent protease inhibitors, such as ritonavir and lopinavir, were further refined to enhance efficacy and reduce adverse effects, significantly improving the treatment outcomes for patients with HIV/AIDS.
Key Takeaways for Step III
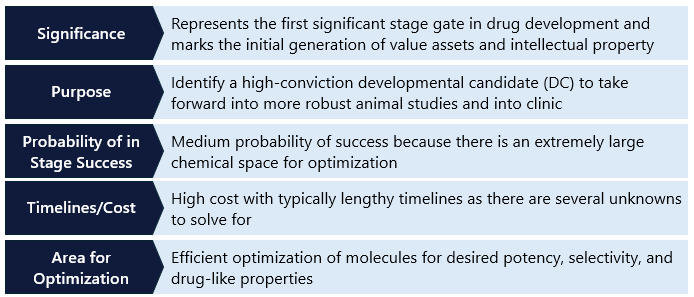
Step 4. IND Enabling Studies
After the lead optimization phase, promising drug candidates enter preclinical development. This critical stage involves extensive testing to evaluate the drug candidate’s safety, efficacy, and pharmacokinetic properties before it progresses to clinical trials. IND-enabling work includes both in vitro and in vivo studies, providing a comprehensive understanding of the drug’s potential effects on biological systems. Though many of the approaches are similar to those used in lead optimization, they are typically much larger and longer studies done in cells and animals to understand the behavior of the drug holistically. These studies form the foundation for a data package submission to the FDA. FDA approval is required before a candidate can begin human testing in the US. Other countries have similar standards (Figure 5).
In Vitro Studies
- Testing on Cell Cultures: In vitro studies involve testing drug candidates on cell cultures to assess their efficacy and toxicity. Researchers use various types of cells, including human cells, to observe how the drug interacts with its target and to identify any cytotoxic effects. This step is crucial for determining the drug’s initial efficacy and for screening out compounds with high toxicity levels early in the process.
- ADME Studies: Absorption, Distribution, Metabolism, and Excretion (“ADME”) studies are essential in understanding how the drug behaves in the body. These studies help predict the drug’s bioavailability, distribution throughout the body, metabolic pathways, and excretion. ADME profiling ensures that the drug reaches its target in sufficient concentrations and has an appropriate half-life, minimizing the risk of adverse effects.
In Vivo Studies
In vivo, studies involve testing drug candidates in animal models to evaluate their safety and efficacy in living organism live mammals spanning from mice to non-human primates (new world monkeys). These studies provide critical insights that cannot be obtained from in vitro experiments alone. For example, dog and rat models are commonly used to understand the safety profile and how the drug is metabolized in the body. These models help researchers understand how the drug affects the entire organism and identify potential side effects.
Real-World Example: IND Enabling Testing of Monoclonal Antibodies
A notable real-world example of IND-enabling development is the testing of infliximab, a monoclonal antibody for autoimmune diseases. Engineered to target specific antigens involved in disease processes, infliximab underwent rigorous preclinical testing to ensure its safety and efficacy before reaching clinical trials. Cell-based in vitro studies confirmed its ability to neutralize tumor necrosis factor-alpha (TNF-α), a cytokine involved in inflammatory responses. Researchers developed a predictive mouse model of arthritis and tested whether these arthritic mice would show slowed or halted disease progression when treated with infliximab.8 These in vivo studies demonstrated infliximab’s efficacy in reducing inflammation and preventing disease progression without significant toxicity.9 These animal studies showed that infliximab could effectively reduce symptoms and inflammation markers, providing clear evidence of its therapeutic potential. The robust preclinical results supported advancing infliximab into clinical trials. This eventually led to its approval and widespread use in treating various autoimmune diseases.
Figure 5: Overview of the components for an IND Data Package.
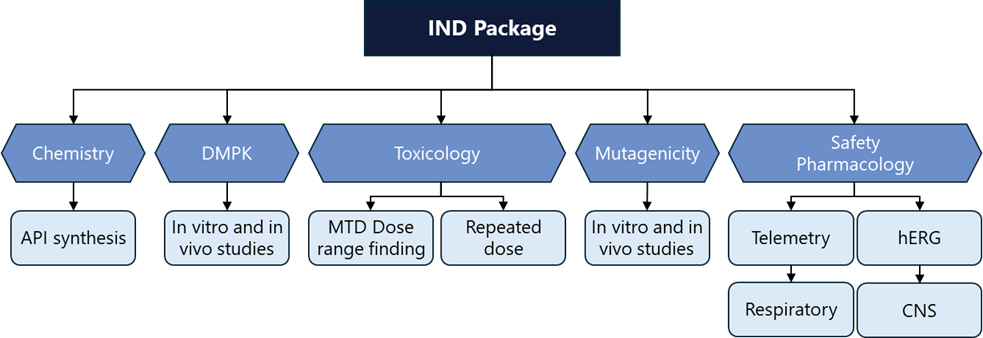
Source: Adgy Life Sciences.
Key Takeaways for Step IV
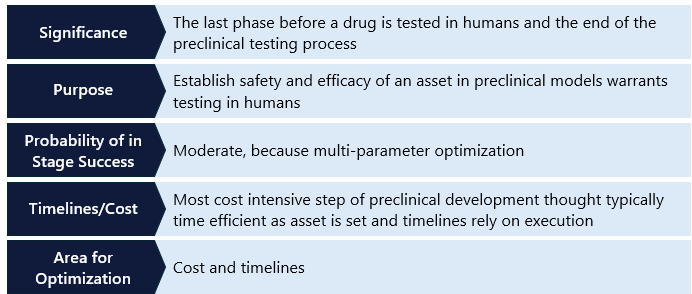
Clinical Development will be covered in Part 2 of the primer series
Current Challenges in Drug Discovery
High Attrition Rates: One of the most significant challenges in drug discovery is the high attrition rate. The journey from initial discovery to an approved drug is fraught with obstacles, and many promising candidates fail during development. This failure can occur at any stage, from preclinical testing to late-stage clinical trials, often due to unforeseen toxicity, lack of efficacy, or poor pharmacokinetics. Even though many of the individual stages have high percentages, the communicative attrition rates result in>30% of drug candidates that enter clinical, and only 5-10% of these drug trials ultimately receive FDA approval. Still, depending on the stage at which clinical challenges emerge, the relevant company could have spent hundreds of millions of dollars.10 It may require further investment to remediate the challenges, or the endeavor could fail altogether. This means that any successful product’s commercial value must be high enough to offset the cost of any failures.
High Cost of Research: Over the past 75 years, drug development costs have continuously escalated. This phenomenon, humorously dubbed Eroom’s Law, reflects an inverse trend to Moore’s Law for microchip development.11 Eroom’s Law observes that the cost of drug development roughly doubles every year, in contrast to Moore’s Law, which states [that the number of computations a semiconductor can complete, per unit, etc etc.]. As a result, the barriers companies face in bringing a drug to market has continued to escalate. All else equal, we view this as one of the factors that continues to support high average returns on capital to the biotech venture capital industry; demand for capital continues to increase at a time in which this specialized form of capital remains scarce.
Figure 6: Eroom’s Law depicted by illustrative historical regression of significant increase of drug discovery and development.
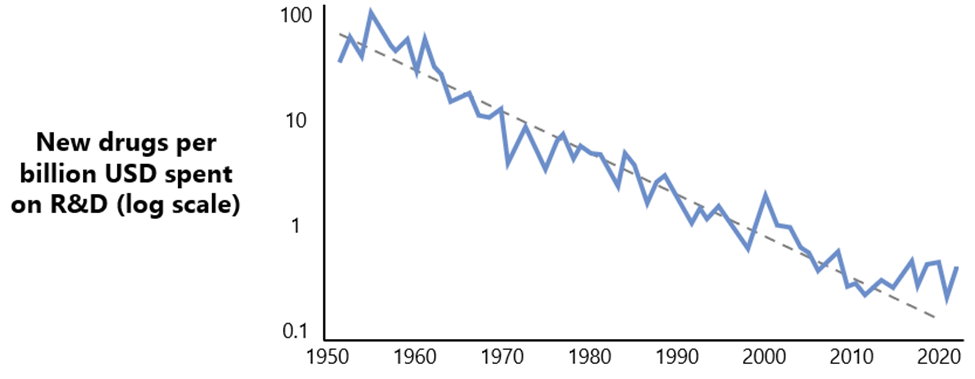
Source: Benchling.
Valley of Death: Significant requirements for capital and time asymmetries exist between value generation and risk reduction in drug development. Combined with the high costs and binary nature of outcomes, this necessitates that companies raise or spend substantial capital to mitigate risks during the development process. These gaps contribute to the “valley of death,” a critical phase where companies may lack the necessary funds to navigate lengthy and expensive stages of development, potentially forcing them to halt their programs (Figure 7). For instance, the biotech company Epizyme faced considerable financial challenges in advancing its cancer drug, Tazemetostat, through clinical trials but overcame these issues to be approved and is now commercially available. Despite promising early results, the high costs and extended timelines required significant capital, leading to delays and funding gaps that exemplify the difficulties of the “valley of death” in drug development.
Figure 7: Illustration of the drug discovery valley of death.
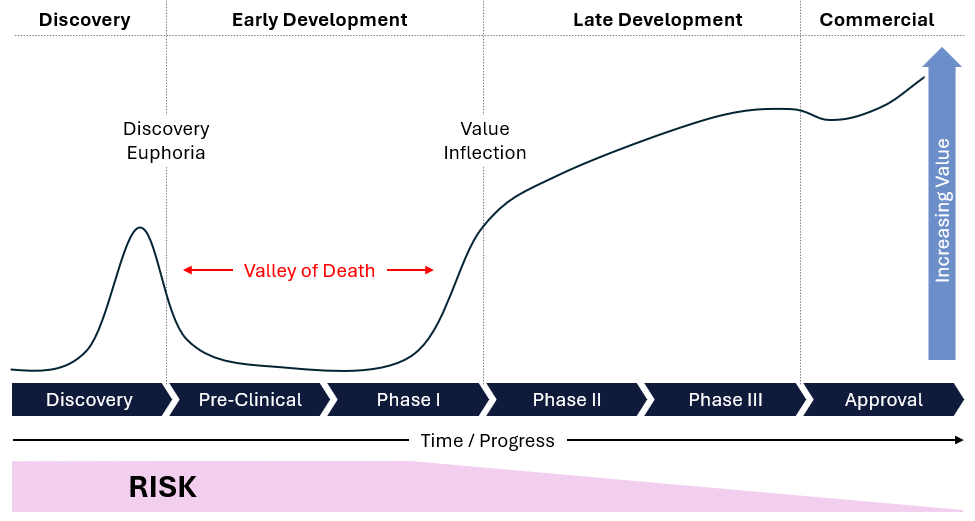
Source: Research gate.
Future Directions in Drug Discovery
Artificial Intelligence (AI): AI is revolutionizing drug discovery by enhancing the ability to predict, analyze and understand complex biological data. The integration of AI in drug discovery has the potential to significantly reduce the time and cost involved in bringing new drugs to market. Here are several ways AI is transforming this field:
- Predicting Protein Structures: One of the most notable applications of AI in drug discovery is predicting protein structures. Accurate prediction of protein folding is crucial for understanding how drugs interact with their targets. DeepMind’s AlphaFold has made significant breakthroughs in this area. AlphaFold’s ability to predict the three-dimensional structures of proteins with high accuracy has provided researchers with vital insights into the fundamental mechanisms of diseases. This advancement accelerates the identification of new drug targets and designing molecules that can interact precisely with these targets.
- Drug Design and Optimization: AI algorithms are being used to design and optimize drug candidates. Machine learning models can analyze large datasets of chemical compounds and their biological activities to predict which compounds will have the desired effects on specific targets. This process can save millions of dollars and accelerate the process in the lead optimization step by year. For example, Insilico Medicine utilized AI to identify new drug candidates for fibrosis, significantly speeding up the discovery process. AI-driven platforms can generate novel compounds, optimize existing ones, and predict their pharmacokinetic and toxicological properties, streamlining the drug design phase.
- Virtual Screening: AI enhances initial screening by virtually predicting which compounds are most likely to bind to a target based in the hit to lead stage. Traditional virtual screening methods can be time-consuming and computationally intensive, but AI can process vast libraries of compounds more efficiently. Companies like Atomwise use AI to conduct initial virtual screenings, identifying potential drug candidates in a fraction of the time required by conventional methods. This approach increases the likelihood of finding effective drugs and reduces the reliance on high-throughput physical screening.
- Biomarker Discovery: Identifying biomarkers is essential for developing targeted therapies and companion diagnostics. These biomarkers are samples from the patient that can help understand the disease progression and how well the patient is reacting to therapy. AI can analyze complex biological data, such as genomics, proteomics, and metabolomics, to identify potential biomarkers that indicate how a patient will respond to a particular treatment. IBM Watson for Drug Discovery, for example, uses AI to uncover hidden connections in biomedical data, aiding in the discovery of new biomarkers and therapeutic targets.
- Predicting Clinical Trial Outcomes: AI models can predict the outcomes of clinical trials by analyzing historical trial data and identifying factors that influence success rates. This predictive capability helps in designing more effective trials, selecting the right patient populations, and optimizing protocols. AI-driven predictive analytics can reduce the risk of trial failure, saving time and resources.
We recently published a whitepaper on the impact of AI on healthcare, which you can read here.
Novel Therapeutic Modalities: The development of novel therapeutic modalities, including gene therapy, CRISPR and biologics, is opening new frontiers in medicine. Gene therapy aims to treat or prevent diseases by introducing, removing, or altering genetic material within a patient’s cells. An example is the FDA-approved gene therapy Luxturna, which treats a rare inherited vision loss. CRISPR technology, which allows precise genome editing, holds promise for treating genetic disorders by correcting mutations at their source. Biologics, such as monoclonal antibodies and cell-based therapies, offer targeted treatment options for a wide range of diseases, including autoimmune disorders and cancers.
Real-World Examples
AI in Drug Discovery: Exscientia was an AI-driven drug discovery company. In 2020, Exscientia collaborated with Sumitomo Dainippon Pharma to develop DSP-1181, a drug candidate for treating obsessive-compulsive disorder (OCD). Using its AI platform, Exscientia was able to design the drug in just 12 months, a process that typically takes 3 to 5 years using traditional methods. The AI system efficiently analyzed and processed vast amounts of data to identify and optimize the drug candidate, demonstrating a significant acceleration in the drug discovery process. Specifically, they used AI to rapidly predict new chemical structures during lead optimization, significantly faster than the traditional method DSP-1181 advanced to clinical trials, highlighting the potential of AI to speed up the development of new medications.12 Exscientia entered an agreement to be acquired by Recursion Pharmaceuticals in August 2024.
CRISPR and Gene Therapy: The application of CRISPR technology in clinical settings is exemplified by ongoing trials for sickle cell disease and beta-thalassemia. Companies like CRISPR Therapeutics and Vertex Pharmaceuticals are leading the way with CRISPR-based treatments that aim to edit the faulty genes responsible for these conditions. Early results have shown promise, with some patients achieving significant improvements, highlighting the potential of gene editing as a therapeutic modality.
Precision Autoimmune Diseases: The development of biological therapies, such as adalimumab (Humira) and infliximab (Remicade), has pioneered the treatment of autoimmune diseases like rheumatoid arthritis and Crohn’s disease. These drugs specifically target inflammatory pathways, offering relief to patients who do not respond to traditional therapies. A significant challenge in treating autoimmune conditions is the ability to precisely target disease cells or tissues while avoiding toxicity in healthy ones. Recent biological insights have enabled the adaptation of clinically derisked technology from precision oncology for autoimmune applications. Luma recently published a white paper highlighting advancements and opportunities in this innovative field. The success of these biologics underscores the importance of targeted treatments in managing complex chronic conditions.
Concluding Thoughts
Drug discovery is not for the faint of heart. It requires unwavering conviction, passion, perseverance, a vast team of dedicated individuals, and substantial financial resources to bring a therapy to market. Despite these challenges, countless people in our sector commit decades, even entire lifetimes, to the hope of developing a drug that can offer patients a better life than their disease allows today. Although the journey can be daunting, it is profoundly rewarding to know that all this effort, time, and investment can significantly impact patients’ lives and improve global health within our lifetimes.
- https://www.forbes.com/sites/matthewherper/2013/08/11/how-the-staggering-cost-of-inventing-new-drugs-is-shaping-the-future-of-medicine/#7753cc096bfc ↩︎
- https://www.biospace.com/article/releases/pharmaceutical-market-size-to-hit-around-usd-2-832-66-bn-by-2033/ ↩︎
- https://www.biospace.com/article/releases/pharmaceutical-market-size-to-hit-around-usd-2-832-66-bn-by-2033/ ↩︎
- https://web.archive.org/web/20150905144824/https://dtp.nci.nih.gov/timeline/flash/success_stories/S2_Taxol.htm ↩︎
- https://www.nature.com/scitable/topicpage/gleevec-the-breakthrough-in-cancer-treatment-565/ ↩︎
- https://www.mdpi.com/1422-0067/23/22/14178 ↩︎
- https://link.springer.com/chapter/10.1007/978-981-16-5180-9_16 ↩︎
- https://www.ncbi.nlm.nih.gov/pmc/articles/PMC453150/ ↩︎
- https://www.sciencedirect.com/science/article/abs/pii/016158909390106L ↩︎
- https://link.springer.com/article/10.1007/s43441-023-00509-1 ↩︎
- https://www.nature.com/articles/nrd3681 ↩︎
- https://www.sumitomo-pharma.com/news/20200130.html ↩︎