The AI revolution’s impact on society, particularly healthcare, is set to surpass the transformative effects of the Industrial and Information Revolutions. Real-world evidence of this can be seen in the application of AI-driven diagnostics and therapeutics, which have significantly enhanced the accuracy and speed of disease detection and rate of targeting and curing disease. The forthcoming role of AI in healthcare is ambitious yet achievable but requires a level of collaboration, innovation, and dedication to a brighter and healthier future.
April 2024
To read the scientific addendum to this whitepaper, click here.
The journey from a research lab to a patient for a single drug spans an average of 12 years. The accompanying cost is by some estimates over $2 billion. Even still, the odds of success remain minuscule, standing at one in 5,000.1,2 The biotechnology industry, in its current state, appears inefficient; however, the integration of artificial intelligence (“AI”) with exemplary scientific practices presents a promising avenue for significant improvement.
The advent of AI models and applications has sparked a wave of breakthroughs, culminating in the creation of $6 trillion in market capitalization for the “Magnificent 7” stocks alone since the launch of ChatGPT (Figure 1).
Figure 1: The “Magnificent 7” stocks – Microsoft, Amazon, Meta, Apple, Google parent Alphabet, Nvidia and Tesla – drastically outperformed the major U.S. indexes in 2023 as the market recognized them as AI winners. Outperformance YTD has continued with more innovation in the AI space expected in 2024.
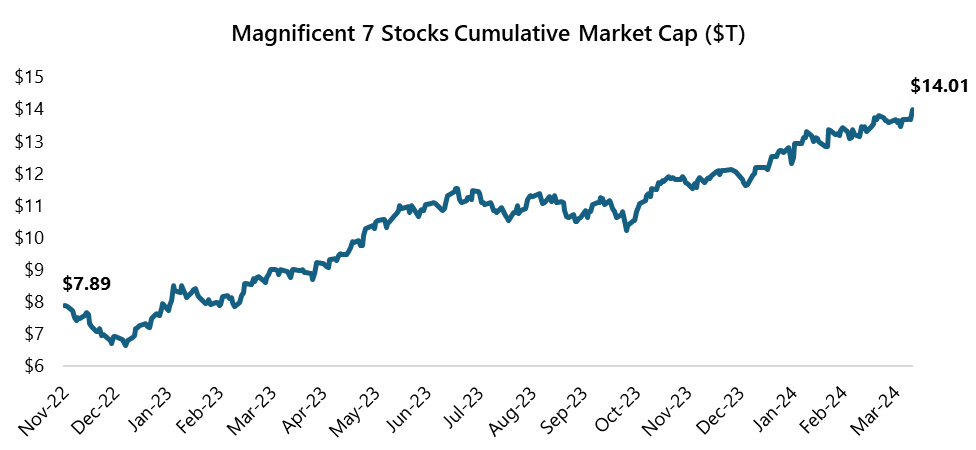
Source: Public market data as of April 10, 2024.
Unlike tech stocks, AI’s impact is not yet baked into healthcare valuations. AI has already begun generating new ideas, discoveries and potential opportunities to accelerate the healthcare sector to become more efficient. We can observe this trend by looking at the proliferation of AI mentions in scientific publications and healthcare AI expenditures (Figure 2). The healthcare industry spent approximately $13 billion on AI hardware and software in 2023, with projections to rise to $47 billion by 2028.3 CB Insights reports that between 2019 and 2022, investors injected $31.5 billion in equity funding into AI healthcare ventures.
Figure 2: The number of Pubmed abstracts with the keywords “Machine Learning” or “Artificial Intelligence” has increased dramatically over the past 15 years.
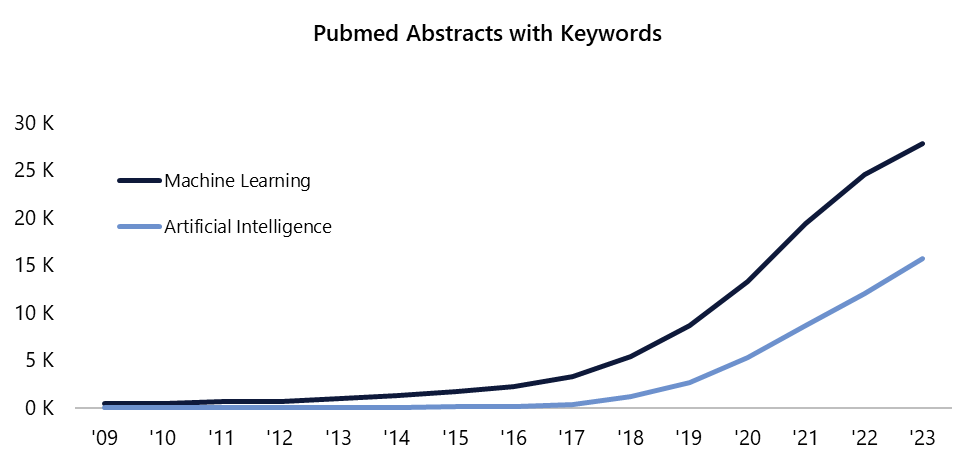
Source: Pubmed.
Broadly on AI: what is it, and why should I care?
AI, in its essence, encompasses the development of computer systems that can perform tasks that typically require human intelligence. These tasks include learning, decision-making, language understanding, and visual perception. The dawn of AI marks a significant leap forward in our ability to process information, automate complex processes and solve intricate problems across almost all sectors and industries. The potential societal impact of AI cannot be overstated. These technologies promise to revolutionize every sector of the economy, from manufacturing to healthcare, education to finance, by enhancing efficiency, unlocking new insights and opening avenues for innovation that were previously unimaginable.
At the core of recent advancements in AI are neural networks inspired by the human brain’s method of processing data inputs and generating predictive outputs. These networks, essential to AI’s evolution, frequently derive their architecture from biological structures, particularly the brain’s complex neural pathways. This biomimetic approach is far from being merely symbolic; it’s fundamentally practical, equipping AI systems to analyze and understand complex information similarly to how biological entities do. Moreover, the deployment of AI in biological contexts provides crucial insights that feedback into AI development, refining algorithms to better emulate natural neural processes. This continuous feedback loop not only drives technological advancements in healthcare but also enhances our comprehension of the biological inspirations behind these AI innovations.
AI’s impact on society and healthcare: shaving billions of dollars and years off the quest to save lives
The AI revolution’s impact on society, particularly healthcare, is set to surpass the transformative effects of the Industrial and Information Revolutions. Real-world evidence of this can be seen in the application of AI-driven models, e.g. Google DeepMind’s AlphaFold, to significantly enhance the speed of drug development.
As with many scientific breakthroughs, Google’s foray into AI within healthcare seems almost serendipitous. Initially, after Google’s DeepMind demonstrated remarkable proficiency in video games, the company decided to leverage its AI capabilities for more academic applications, such as healthcare. This backbone model was used to develop AlphaFold, an AI that predicts a protein’s 3D structure from its amino acid sequences (Figure 3). This is important because there are over 200 million known proteins, each with a unique 3D shape that determines how they work and what they do. Before AlphaFold, scientists could not accurately determine a protein’s unique 3D shape without millions of dollars and years of trial and error. Google taught AlphaFold to predict structures by showing it the sequences and structures of ~100,000 known proteins. Now, AlphaFold has predicted structures for an estimated 98.5% of human proteins with over 92.4% accuracy in positioning atoms, matching experimental methods like X-ray crystallography.2 Better yet, AlphaFold’s predictions are freely available to the entire scientific community. This represents a massive leap forward in the quest to generate research tackling disease and finding new medicines. For one, AlphaFold can accurately detect over 50 eye diseases from 3D scans.4 This offers a glimpse into a world where early detection and treatment of diseases become the norm rather than the exception.
DeepMind is just one example underscoring AI’s ability to navigate the complexities of biology and healthcare data, translating into life-saving applications and fundamentally changing medical care approaches. Importantly, AI work is just starting; over half of the current 1,500 health AI vendors were established in the last seven years.
Figure 3: Determining protein structure from amino acid sequence.
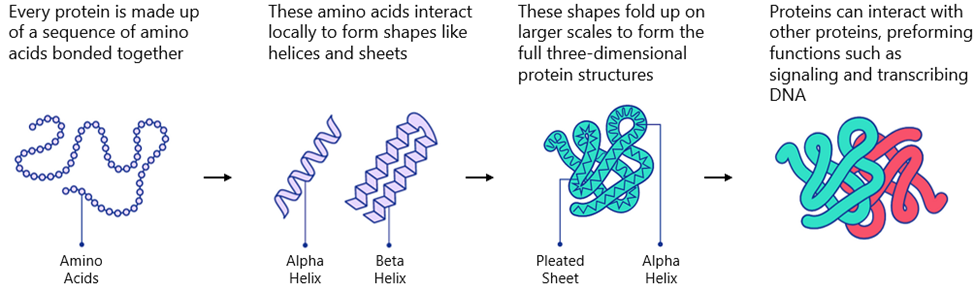
Source: Baker Lab.
The inherent complexity of biology: the AI revolution will likely play out differently across different areas of healthcare, and data is king
Though Google could (relatively) easily apply its video game AI to biology in just four years, the application of AI in healthcare presents a unique set of challenges, starkly contrasting the relatively straightforward applications seen in other sectors. These challenges primarily stem from the intricate complexity of biological systems and the formidable task of obtaining robust, accurate, and comprehensive data in biology. Unlike the datasets used in finance and marketing, where variables are often well-defined and quantifiable, biological data encompasses many variables that interact in complex, usually unpredictable ways. This intrinsic complexity makes modeling biological systems a daunting task for AI.
One of the foundational hurdles in applying AI to healthcare is the heterogeneity and multidimensionality of biological data. Genetic, environmental and lifestyle factors influence human health and disease, each contributing to the overall complexity of biological systems. For instance, a single genetic mutation can have vastly different effects in different individuals (known as genetic penetrance), depending on the context of thousands of other genes and a myriad of environmental exposures and lifestyle choices. This level of complexity requires AI models to process vast amounts of data and understand and predict the interactions between these variables. This task remains at the frontier of current capabilities.
Moreover, obtaining high-quality, comprehensive biological data poses another significant challenge. Much of the data in healthcare is fragmented across different systems, encoded in unstructured formats or locked within the proprietary databases of research institutions and private companies. Privacy concerns and ethical considerations further complicate data collection, limiting access to the broad datasets necessary for training robust AI models. Even when data is available, it often needs more diversity and representativeness to ensure that AI-driven interventions are effective and equitable across different populations. Even with these limitations, groups like the Chan Zuckerberg Initiative and the Allen Institute are working on collecting robust and well-curved data to help train new models for applications in multiple diseases.
The dynamic nature of biological systems adds another layer of complexity. Unlike static datasets, biological data can change over time, with disease progression, treatment interventions, and lifestyle changes introducing continuous variability. Therefore, AI systems in healthcare must be capable of analyzing static snapshots of data and understanding and predicting how these data points evolve over time, a challenge that requires sophisticated modeling techniques and continuous learning capabilities.
Despite or, better yet, because of these challenges, the potential rewards of applying AI in healthcare are immense. AI has the potential to revolutionize diagnostics, personalized medicine and treatment planning, making healthcare more predictive, personalized and preventive. Overcoming the unique challenges posed by the complexity of biological systems and the difficulty of obtaining robust and accurate data will require innovative approaches to AI model development, data collection and interdisciplinary collaboration between technologists, biologists and clinicians. The journey towards fully realizing AI’s potential in healthcare is complex. Still, the great strides in this direction are promising, signaling a future where AI-driven insights lead to better health outcomes for all.
Great science alone isn’t enough: AI is necessary to continue advancing healthcare
In the dynamic landscape of modern business, adopting AI has transcended from a mere competitive edge to an indispensable necessity. This assertion holds particularly true for the healthcare sector, where AI’s integration promises to refine existing processes and workflows and redefine the paradigms of patient care and medical research. The economic benefits of AI in healthcare are significant; as noted, the healthcare industry is projected to spend $47 billion on AI hardware and software by 2028. This represents a lucrative opportunity for investors and entrepreneurs looking to expand on the AI revolution in healthcare.
AI’s ability to process and analyze data at a scale and speed unattainable by human capabilities alone has led to significant advancements in diagnostics, patient care, drug development and operational efficiencies. For instance, Philips developed the eICU program, which uses AI to aggregate and analyze patient data from ICUs to provide insights into medical decision-making, resulting in a 23% reduction in mortality rates across all four hospitals studied. Furthermore, the average length of stay for a patient in the ICU dropped by 49%, while lengths of stay throughout the health system decreased by 35%.5 In drug discovery and development, AI expedites the identification of potential therapeutic targets and predicts the efficacy of drug candidates, dramatically reducing the time and cost associated with bringing new drugs to market.
Beyond these immediate applications, AI facilitates a more personalized approach to healthcare, tailoring treatments and health plans to the individual’s unique genetic makeup and lifestyle factors. This shift towards customized medicine enhances the effectiveness of treatments. It represents a significant leap towards preventive healthcare, potentially saving costs and lives by addressing conditions before they escalate into more severe problems. However, the necessity of AI in healthcare extends beyond improving patient outcomes and operational efficiencies; it is also crucial for the sustainability and growth of businesses within this sector. In an environment where regulatory pressures, rising costs and increasing demands for high-quality patient care converge, AI provides the tools to navigate these challenges effectively. By automating routine tasks, AI allows healthcare professionals to focus on more complex and nuanced aspects of patient care, thereby enhancing patient outcomes. Furthermore, AI’s predictive capabilities enable healthcare organizations to make more informed strategic decisions, from resource allocation to market expansion strategies.
In essence, embracing AI in healthcare is not merely an option for forward-thinking organizations but a strategic imperative for all. Businesses that employ the power of AI will not only lead to innovation and efficiency but will also set the standard for patient care in the future. As AI continues to evolve and its applications within healthcare expand, the gap between adopters and non-adopters will widen, underscoring the urgency for all businesses in this sector to integrate AI into their operations. The go-forward success of healthcare businesses will increasingly depend on their ability to leverage AI technologies to meet the demands of a rapidly changing world, making the case for AI’s necessity not just compelling but categorical.
“Garbage in, Garbage out”: the quality and availability of omics data are critical factors to advancing AI in healthcare
The accuracy and power of AI model predictions, patterns, structures or generative outputs hinge critically on the quality of the input data, bringing the old modeling adage “Garbage in, Garbage out” into sharp relief. While the speed and breadth of applications that AI models have achieved is undeniably impressive, it’s crucial to recognize that the value of data and access to datasets is poised to soon surpass the value of the models. This insight is particularly relevant in the revolution currently underway in healthcare, driven by omics data – genomics, proteomics, metabolomics and more. This revolution is reshaping our understanding of disease, treatment, and wellness, as integrating these omics data types offers a comprehensive view of the biological underpinnings of health, thus paving the way for personalized medicine and targeted therapies.
However, the availability of these omics datasets and methodologies will significantly influence the landscape of healthcare innovation and access. The potential scenarios of a) widespread public availability, b) high restriction or c) a balanced approach to data access each illustrate varying impacts on healthcare progress, underscoring the intertwined future of AI models and omics data in defining the next frontier in healthcare.
- Scenario A: omics data is democratized, likely leading to an unprecedented acceleration in healthcare innovation – With scientists, researchers and clinicians worldwide having open access to vast datasets, the collaborative potential for discovering new disease mechanisms, therapeutic targets and treatment modalities would expand exponentially. This openness could also foster a more inclusive research environment, where even institutions with limited resources could contribute to and benefit from global scientific advancements. This is the type of open collaboration fostered by AlphaFold. It was built on publicly available datasets, and the AI model itself was made publicly accessible for anyone to use. Moreover, initiatives have been undertaken to standardize and curate massive genomic datasets, such as those by The Cancer Genome Atlas (“TCGA”), and the UK Biobank Proteomics and other datasets. All of these efforts have taken decades – and the dedication of tens of thousands of researchers – and still require massive curation and maintenance to generate effective AI model outputs.
- Scenario B: omics data access is tightly controlled by select entities, potentially leading the deployment of AI to become a double-edged sword – On one hand, AI could streamline research and development within these privileged circles, pushing the boundaries of proprietary medical breakthroughs. On the other hand, this restriction could limit AI’s learning potential by confining it to siloed datasets, potentially slowing the pace of innovation and widening the gap in healthcare access. Given the value of these datasets, we have already seen private entities begin to hoard data for commercial purposes or continue to restrict access to proprietary datasets to maintain a competitive edge. Companies like Flatiron Health and Tempus have been gathering massive datasets for commercialization purposes, while almost all therapeutic companies restrict the publication of their internal datasets to maintain trade secrets and competitive advantage.
- Scenario C: a middle-ground, balanced approach to omics data availability has the highest likelihood of unfolding in the near future – There will likely be significant academic and government-funded generation and curation of large omic datasets, with private companies restricting access to proprietary datasets. Over time, these large public datasets will mature and expand to include new omics datasets, and standardization will likely play a crucial role. Large initiatives like the Chan Zuckerberg Initiative are already building databases with the scale and infrastructure necessary to generate high-quality datasets. Private companies will likely release data after it has been depleted of value or when there is greater value in sharing the data than in hoarding it. We have already seen small biotechs like Leash Bio adopt an open-source approach and share data with the strategy that more integration and data will only enhance their efforts to develop novel therapeutics.
Venture capital’s role in driving Healthcare x AI forward
From the vantage point of investment in the rapidly evolving landscape of healthcare, the integration of AI stands out not just as an opportunity but as a critical imperative. Investors increasingly recognize that the future healthcare leaders will be those organizations that adeptly harness AI to drive innovations, improve patient outcomes, and streamline operations. In contrast, companies that need to be faster to adopt AI technologies risk falling behind and potentially facing obsolescence. The stark reality is that the healthcare sector is at a pivotal inflection point where the adoption of AI can significantly differentiate between market leaders and laggards. These dynamic underscores the critical importance of investment strategies that prioritize and support the integration of AI in healthcare.
The argument for prioritizing investments in AI-enhanced healthcare companies is multifaceted. Firstly, AI’s ability to process and analyze vast datasets at speeds and accuracies unattainable by human capability transforms diagnostics, treatment personalization, and patient monitoring. This technological prowess is pivotal in decoding complex health data, leading to breakthroughs in understanding disease mechanisms and developing targeted therapies. For investors, backing companies that leverage AI in these areas offers the promise of substantial financial returns and the opportunity to contribute to significant societal impacts in terms of improved health outcomes and longevity.
Furthermore, the synergy between great science and AI utilization forms a cornerstone for the next generation of healthcare innovation. AI does not operate in a vacuum; its most significant potential is unlocked when combined with cutting-edge scientific discoveries and insights. Companies that cultivate a culture of innovation, where AI tools are used to accelerate and expand the impact of scientific research, are poised for success. This fusion of AI with scientific inquiry creates new frontiers in personalized medicine, making treatments more effective and reducing adverse outcomes. From an investment perspective, this represents fertile ground for growth, as companies at the forefront of this convergence are likely to be in charge of introducing novel healthcare solutions and capture significant market share.
Investors, including us at Luma Group, are encouraged to scrutinize the current AI capabilities of healthcare companies and their potential to innovate and adapt. The criteria for investment decisions should include an evaluation of a company’s commitment to AI integration, the robustness of its data infrastructure, the caliber of its scientific research, and its capacity for leveraging AI to translate scientific discoveries into clinical applications. Companies that demonstrate a clear vision for integrating AI with scientific exploration are the ones that will navigate the complexities of modern healthcare, delivering solutions that address unmet medical needs and driving the future of medicine.
One of our key focus areas here at Luma Group is investing in AI-driven enterprises pioneering drug discovery and diagnostics advancements. Among our investments is Rome Therapeutics, which delves into large human genetics datasets to explore the “dark genome”—a segment historically dismissed as evolutionary junk, with no coding for proteins or known biological function. Rome Therapeutics has discovered novel therapeutic targets for autoimmune diseases hidden within these previously overlooked genomic territories through its innovative AI models. Their AI approach examined the vast dark genome region to identify regions highly expressed in patients with specific autoimmune diseases. Within these regions, they discovered nonconical protein expression patterns and found ancient retroviral proteins that are driving the disease progression. This breakthrough could not have been achieved without the sophisticated analysis enabled by their AI technology.
Our recent investment Curve Biosciences is a trailblazer in diagnostics focusing on developing cutting-edge early-stage liver diagnostics that will leverage AI. Curve’s data engine analyzes methylation patterns in cell-free circulating DNA in the blood. While it is understood that total methylation levels escalate over time, the exact tissue methylation patterns distinguishable in cell-free DNA that strongly relate with disease progression have remained elusive. Through the application of AI to analyze vast patient tissue DNA methylation datasets across various stages of chronic disease progression, Curve Biosciences has pinpointed predictive methylation signatures with subtle, previously undetectable patterns of methylation that strongly correlate with the stage of and progression of the disease (Figure 4). This innovative approach exemplifies the power of AI in transforming diagnostics and how we understand disease mechanisms with an immediate application in diagnostics.
Figure 4: Curve leverage AI/ML to process large PCR, microarray, and sequencing data sets to inform their screening technology.
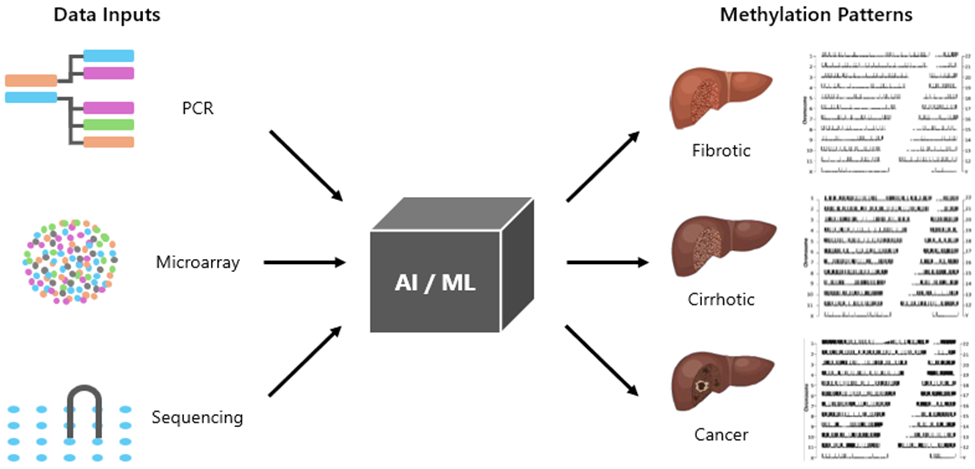
Source: Company Materials.
The investment perspective on AI in healthcare is clear: leveraging AI is not merely an option but a strategic imperative for success. As the sector continues to evolve, the combination of innovative science and AI utilization will distinguish the leaders from the followers. For investors, the message is equally clear: support and invest in companies that understand and act on this imperative, for they are the ones that will shape the future of healthcare, delivering both substantial financial returns and transformative improvements in human health outcomes.
Call to action
In the vast and ever-evolving landscape of healthcare, where the promise of groundbreaking innovation stands on the horizon, a profound opportunity exists to reshape healthcare and make meaningful improvements in human health. The forthcoming role of AI in healthcare is ambitious yet achievable but requires a level of collaboration, innovation, and dedication to a brighter and healthier future.
Looking ahead, AI’s influence on healthcare will only broaden as the technology itself advances. At some point in the not-so-distant future, we anticipate that the advent of Advanced General Intelligence (“AGI”) could lead to a whole new era in healthcare by surpassing human intelligence to predict diseases, tailor treatments to individual genetics and automate complex medical procedures. AGI promises to enhance diagnostic accuracy, optimize healthcare management and improve resource allocation, fundamentally increasing the quality and accessibility of care. AI’s path forward in this space will be complex and filled with challenges, yet it is paved with the potential for extraordinary advancements. This will require a collective effort by researchers, clinicians, technologists, entrepreneurs and investors, sharing knowledge, resources and visions to catalyze the development of solutions that can address the most pressing health challenges of our time. Moreover, this collective endeavor demands a commitment to relentless ideation and innovation. We are at the cusp of a new era in healthcare that leverages AI, genomics and emerging technologies to make new insights into human health and leverage them for new therapeutics, clinical trials, diagnostics and other healthcare applications. We believe AI will dramatically improve patient outcomes and redefine healthcare in the
- https://www.pwc.com/gx/en/industries/healthcare/publications/ai-robotics-new-health/transforming-healthcare.html ↩︎
- https://www.biopharmadive.com/news/new-drug-cost-research-development-market-jama-study/573381/ ↩︎
- https://www.economist.com/technology-quarterly/2024/03/27/ais-will-make-health-care-safer-and-better ↩︎
- https://deepmind.google/discover/blog/a-major-milestone-for-the-treatment-of-eye-disease/ ↩︎
- https://www.fiercebiotech.com/medtech/philips-illustrates-gains-over-15-years-a-telehealth-powered-icu ↩︎