The convergence of various factors, including advancements in OMIC data, repurposing of precision oncology technologies and high demand from patients and pharmaceutical companies, has positioned inflammation and immunology sectors for explosive growth, driving the development of safer and more effective precision therapies.
March 2024
Inflammation and Immunology Disease Landscape
Inflammatory and Immunology (I&I) diseases affect approximately 35 million Americans or over 10% of the population. Despite the prevalence, current treatments are ineffective in managing these conditions.
Unsurprisingly, this lack of effective treatments has led to significant demand for new and innovative therapies that are safe and efficient. However, biotech and pharma R&D focus to date has largely been on more severe indications (e.g., oncology), which despite a smaller size, have seen rapid market growth. Precision therapy methods, harnessing established and de-risked technologies derived from precision oncology, hold the promise of catalyzing a comparable revolution in the treatment of I&I diseases. This presents an opportunity for enhanced disease management and ultimately, superior patient outcomes.
The lack of innovation in I&I drug development has been a critical factor contributing to the slower growth of the I&I market. Interestingly, despite the two times larger patient population than oncology, I&I diseases only generate 60% of the market size (Figure 1).
Figure 1: Comparison of I&I disease and oncology markets.
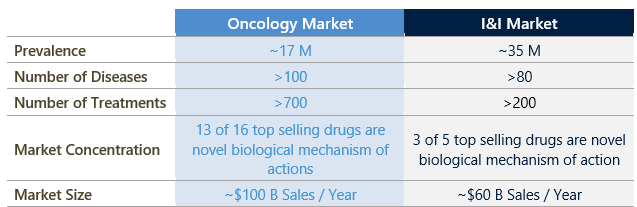
Source: SEER, NIH, IQVIA, Global Data, Evaluate Pharma.
This discrepancy cannot be attributed to differences in treatable disease subtypes or drug treatment costs, but instead to the limited treatment options available for I&I diseases. Limited options have resulted in redundant use cases and lower overall market penetration.
In comparison, oncology has more than twice the number of marketed drugs, which are more diverse in their mechanisms of action and use cases. For example, the top 16 selling oncology drugs generated over $50 billion in sales in 2022 alone according to Global Data, accounting for approximately 50% of the total sales, and 13 out of 16 of these therapies have novel biological mechanisms of action. On the other hand, the I&I market is dominated by only five drugs that comprise around 50% of the market according to Global Data and have only three unique biological mechanisms of action. This dynamic highlights the need for innovation and the potential for market expansion in the space.
The historical safety issues in I&I drug development have been a primary reason for the lag in the market’s growth. Many promising drugs that have demonstrated efficacy in preclinical and clinical trials have failed to enter the market due to safety concerns associated with chronic use. A prime example is CD20 targeting antibodies like Rituxan (rituximab) have shown promising efficacy in the clinic for multiple I&I indications, the class of drugs harbors severe safety issues/risks (opportunistic infection, anaphylaxis, acute coronary syndrome and even death) with chronic use. This “safety Achilles heel” results from target redundancy in disease and healthy cells/tissues, where many tractable drug targets overlap with those expressed in healthy states.
The need for more innovation in addressing these safety concerns has hampered the progress of I&I drug development, with only Stelara (an anti IL-12/IL23 antibodies used for the treatment of Crohn’s disease, ulcerative clotisese, plaque psoriasis and psoriatic arthritis) out of the top five selling I&I drugs entering the market in the past 15 years. This lack of innovation has significantly impacted the growth and potential of the I&I market. However, it highlights a potential opportunity to drive market growth with novel approaches.
Looking forward, I&I drug development can learn from the similar challenges experienced in oncology drug development in the 1990s and early 2000s, which were overcome by shifting to a personalized medicine approach.
Chemotherapy drugs (i.e., antineoplastics) were the legacy standard of care in oncology and have broad mechanisms of action, systemically killing rapidly dividing cells. While this can successfully target cancer, these cells also exist in several non-cancerous tissue types like hair and the lining of the stomach, resulting in the severe side effect profile that is ubiquitous in the class. The non-specific targeting of cells created an immense treatment burden on patients and spurred the improvements in specificity, efficacy and safety seen in the last decade. New scientific insights from collecting and analyzing massive patient datasets enabled this shift, paired with innovative technologies that allowed therapies to target tumor cells while selectively sparing healthy cells. This shift to precision approaches ushered in the era of precision oncology, unlocking enormous market growth that quadrupled in less than 15 years (Figure 2) and vastly improved patient outcomes (Figure 3). I&I drug development can leverage similar strategies to address safety concerns and drive innovation, paving the way for its growth and success in the market.
Figure 2: U.S. oncology sales have increased dramatically over the past 10 years with approvals of immunotherapies (e.g., Keytruda) and other targeted agents (e.g., Tagrisso) driving new sales volume.
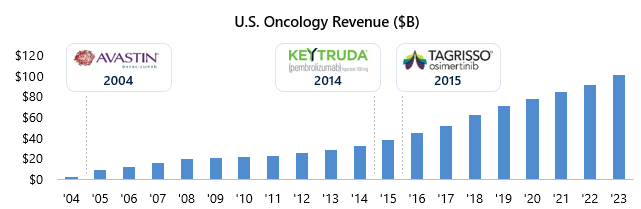
Source: Global Data, Evaluate Pharma.
Figure 3: The growth in the U.S. oncology market was enabled by the dramatic improvements in patient care outcomes supporting their clinical success. This is particularly evident in front line EGFR NSCLC.
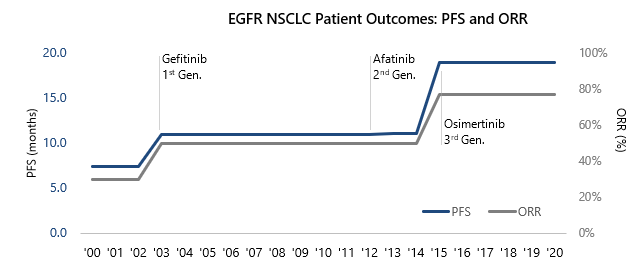
Note: Front line standard of care prior to TKI approvals assumed to be platinum-based chemotherapy. PFS: Progression-free survival, ORR: Overall response rate. Source: FDA label, SEER, clinical trials.
To overcome the innovation bottleneck and thrive in the market, I&I drug development can take cues from the success of oncology by making two fundamental shifts:
- Embracing OMICs Large Data Sets: One crucial missing component in developing I&I precision therapies has been the need for novel biological insights from analyzing massive patient datasets. Until now, researchers have lacked the proper tools, such as single-cell sequencing, advanced fluorescence-activated cell sorting and advanced mass spectrometry, to accurately decipher the immune system’s complexities. By leveraging these technologies and analyzing extensive patient data, I&I drug developers can gain valuable insights into disease mechanisms and identify new targets for precision therapies.
- Repurposing Precision Oncology Technologies: The technologies that revolutionized precision oncology, such as heterobifunctional molecules, bispecific antibodies and cell therapy, can be repurposed for developing precision I&I drugs. In oncology, these innovative technologies have already shown success in targeting tumor cells while sparing healthy cells, and they can also be applied to I&I diseases. By repurposing these proven technologies, I&I drug developers can accelerate the development of safe and effective therapies, overcoming the stagnation in the I&I market.
Investors and companies who focus on analyzing patient data and repurposing precision oncology technologies early on will likely meet the demand for new therapies during the transition to the era of precision therapies in I&I drug development. This success may result in significant therapeutics creation for the large unmet medical need, which are positioned well to gain strong market traction.
I&I Opportunity
Multiple converging factors have positioned the I&I field for rapid growth, potentially surpassing the oncology market. This historically underserved market now has the necessary pieces to overcome historical bottlenecks in I&I drug development and usher in a new era of precision therapies.
One essential advancement is the availability of massive OMIC data, which can be used to address and advance therapeutic development in I&I diseases across several axes. New decoding technologies, like single-cell sequencing, provide novel biological insights for developing precision I&I treatments. This wealth of data has enabled better patient stratification, allowing researchers to characterize patients more accurately and identify responders while excluding non-responders who may pose safety risks. Additionally, this data has revealed new target proteins and cell types that could overcome historical safety issues by increasing drug specificity and expanding the repertoire of potential targets for I&I drug development.
Moreover, I&I drug development can leverage cutting-edge precision oncology technologies that have emerged from the advancements made in the last two decades. These clinically validated technologies, such as adoptive cell therapy and bispecific antibodies, can be repurposed to address safety concerns in I&I drug development. In addition, these modular technologies can be more easily adapted for cost-effective and rapid I&I drug development. For example, companies have repurposed oncology bispecific antibody technology to target immune cells involved in atopic dermatitis while sparing healthy immune cells.
The demand for I&I therapies in the market remains enormous, with many diseases still lacking effective treatments. Many large pharmaceutical and biotechnology players have identified this gap in the market and have been exploring opportunities to access the value. Given their lean and waning I&I drug pipelines, they have looked outwards, lending to several lucrative biotech acquisitions. For instance, companies like Arena Pharmaceutical ($6.7B in 2021), Pandion Therapeutics ($1.85B in 2021) and Momenta Pharmaceuticals ($6.5 billion in 2020) have been acquired by pharmaceutical giants Pfizer, Merck and J&J, respectively, showcasing the appetite for new precision I&I therapies and the potential for continued acquisitions in the future (Figure 4).
Figure 4: Select M&A and licensing I&I deal activity from large cap pharma/biotech since 2020.
Target | Acquirer | Deal Type | Upfront | Total |
Televant | Roche | M&A | $7.1 B | $7.1 B |
Arena Pharmaceuticals | Pfizer | M&A | $6.7 B | $6.7 B |
Momenta Pharmaceuticals | J&J | M&A | $6.5 B | $6.5 B |
ChemoCentryx | Amgen | M&A | $3.7 B | $3.7 B |
Chinook | Novartis | M&A | $3.5 B | $3.5 B |
DICE Therapeutics | Eli Lilly | M&A | $2.4 B | $2.4 B |
Pandion Therapeutics | Merck | M&A | $1.85 B | $1.85 B |
Garcell Biotechnology | AstraZeneca | M&A | $1 B | $1.2 B |
Nimbus Therapeutics | Takeda | License | $4 B | $6 B |
EVQQ Therapeutics | Gilead | License | Not disclosed | $658.5 M |
Source: Company Filings, Pitchbook.
Overall, the convergence of various factors, including advancements in OMIC data, repurposing of precision oncology technologies and high demand from patients and pharmaceutical companies, has positioned I&I for explosive growth, driving the development of safer and more effective precision therapies.
Luma is strategically positioned as a pioneering investor, ready to capitalize on the burgeoning I&I market. We have extensive expertise and active engagement in this market’s two primary value drivers. Additionally, we have an established presence in the emerging field of precision I&I -focused biotech, with active investments in this rapidly growing sector.
Value Driver #1: Luma’s expertise in identifying essential OMICs technology is central to our investment approach and ingrained in our DNA. Our fund has strategically invested in core technologies fueling the new wave of precision I&I therapies. As a result, we possess a keen understanding of the power of OMICs data sets and how to leverage them for the best drug discovery. This knowledge places Luma in a prime position to identify companies and technologies that are highly differentiated and poised for success.
Value Driver #2: Luma’s proficiency in repurposable precision oncology technologies is supported by a deep understanding and proven track record of funding and founding and investing in multiple precision medicine oncology companies. As a result, we are well-versed in cutting-edge technologies like bivalent small molecules, heterobifunctional molecules, bispecific antibodies and others that can be rapidly repurposed to develop precision I&I therapies (Figure 5). This expertise positions us at the forefront of the intersection of precision oncology and I&I, unlocking new possibilities for innovative treatments.
Figure 5: Precision therapeutics modalities with potential for translation into I&I disease.
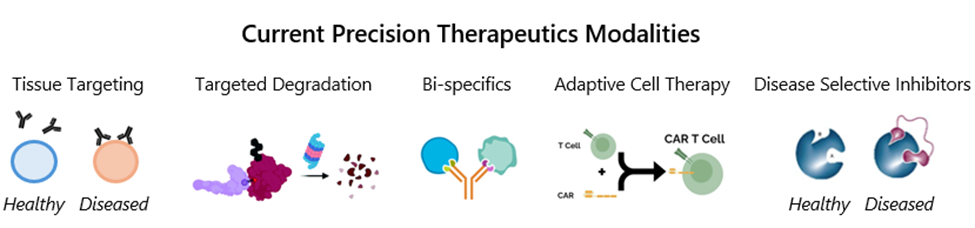
Source: Nature, Cell.
Committed to catalyzing the new precision I&I market, our fund is already actively engaged and primed to capitalize on the new wave of I&I disease market growth. For example, in 2023, we invested in ROME Therapeutics – a company pioneering a new class of precision I&I therapies called LINE-1 reverse transcriptase inhibitors (NRTIs) – demonstrating our proactive approach to identifying and seizing first-mover opportunities.
ROME’s groundbreaking approach targets the uncharted territories of the human genome, often referred to as the ‘dark’ or noncoding regions. Utilizing cutting-edge sequencing and analytical methods, they have made remarkable discoveries. Specifically, they’ve identified certain genomic regions intricately linked to the progression of multiple types 1 interferon-driven I&I diseases, such as Lupus, also referred to as interferonopathies.
Their research has led to the identification of LINE-1 reverse transcriptase (RT) as a new therapeutic target. This target operates upstream of already validated targets, offering a unique approach to treatment (Figure 6). Significantly, it minimizes the risk of infection from opportunistic pathogens, given it is not an immunosuppressant. This promising development is rapidly progressing, and ROME is on track to introduce its first drug to clinical trials in 2024. ROME is applying its approach to advance earlier-stage programs across a spectrum of autoimmune diseases as well as neurodegenerative diseases and cancer.
Figure 6: Rome Therapeutics’ mechanism of action versus approved SLE treatments.
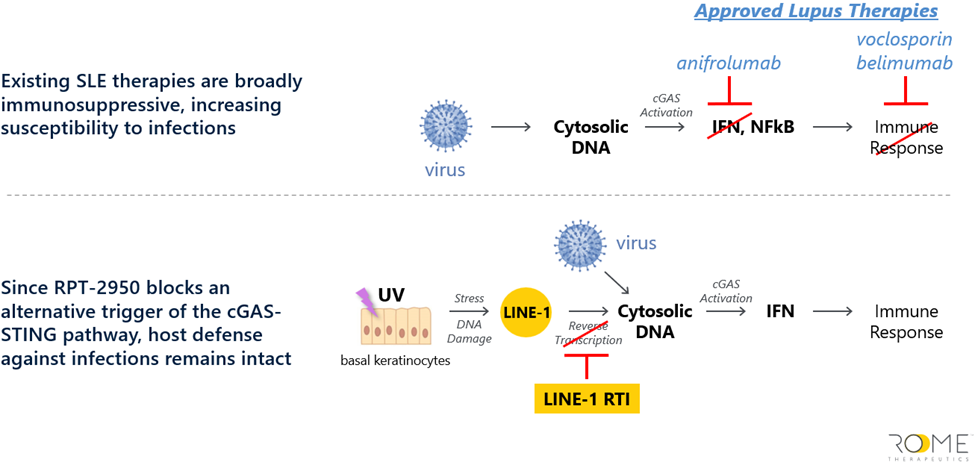
Source: Company materials.
Additionally, we recognize the value in and actively seek investments leveraging precision oncology technologies for I&I therapies, further showcasing our commitment to driving innovation and capturing market opportunities.
Luma is well-prepared to lead the I&I investment landscape, leveraging our expertise in OMICs technology and data, repurposable precision oncology technologies and active participation in pioneering I&I companies.
Conclusion
The I&I market is a rapidly expanding investment sector, but a lack of groundbreaking therapies has hindered its growth. Despite this challenge, the foundational elements are now coming together to propel the development of innovative precision therapies and unlock significant market growth. Luma possesses the expertise, experience and enthusiasm to be a pioneering investor in this promising market. Therefore, we are the ideal investment partner to capitalize on emerging opportunities in the precision I&I market, offering the best growth potential.